Recently, we have presented a paper titled: Bayesian analysis of fracture of polyamide 12 U-notched specimens, in collaboration with the group of Professor Jesús Rodríguez-Pérez (DIMME, Grupo de Durabilidad e Integridad Mecánica de Materiales Estructurales, Escuela Superior de Ciencias Experimentales y Tecnología, Universidad Rey Juan Carlos) in the European Conference of Fracture 2024
We share the Python code in this blog using synthetic notched fracture data as an example of Bayesian non-linear regression.
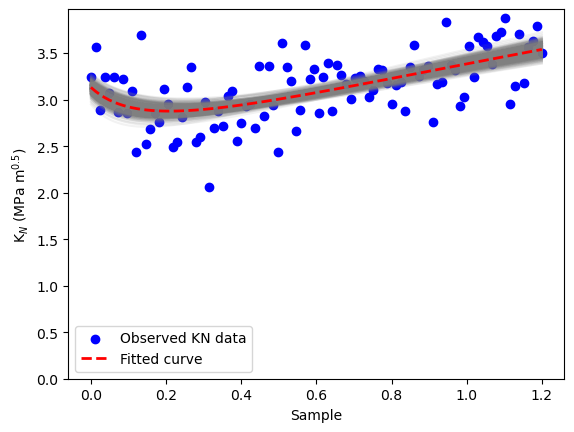
We describe step by step the code used
import numpy as np import matplotlib.pyplot as plt import pymc as pm import arviz as az
def KN_func(KIC, ft, R): pi = np.pi L = (KIC / ft) ** 2 * 1000 KN = KIC * (1 + pi * R / L) ** 1.5 / (1 + 2 * pi * R / L) return KN # Fixed parameters for synthetic data KIC = 3.25 ft = 82.0 # Generate synthetic data N = 100 # Number of data points radio_vect = np.linspace(0, 1.2, N) mean_KN = KN_func(KIC,ft, radio_vect) # Adding noise to simulate real measurements observed_KN_values = mean_KN + np.random.normal(0, 0.3,N) # Plot the synthetic data plt.scatter(radio_vect, observed_KN_values, color='blue', label='Synthetic KN data') plt.plot(radio_vect,mean_KN, color='red', linestyle='--', label='True KN curve') plt.xlabel('Sample') plt.ylabel(r'K$_N$ (MPa m$^{0.5}$)') plt.ylim([0,None]) plt.legend() plt.show()
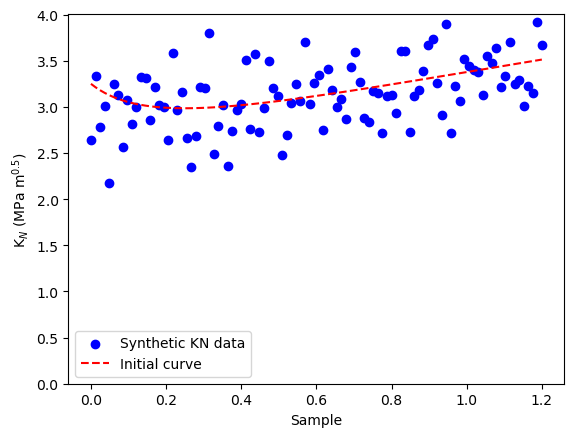
- KIC: Represents the critical stress intensity factor (fracture toughness).
- ft: Critical stress.
- ϵ: The error parameter.
We’ll specify priors for the two material parameters, and, the standard deviation.
with pm.Model() as model: # Priors for KIC, ft, and R based on domain knowledge KIC = pm.Uniform('KIC', 1, 5) ft = pm.Uniform('ft', 10, 150) ϵ = pm.InverseGamma('ϵ', alpha=1.0,beta=1) # Likelihood: observed data is compared against the model function KN_observed = pm.Normal('KN_observed', mu=KN_func(KIC, ft, radio_vect), sigma=ϵ, observed=observed_KN_values) # Inference trace = pm.sample(2000, tune=1000, return_inferencedata=True,cores=3)
Using arviz, a powerful library for Bayesian analysis, we plot the trace of the parameters and their posterior distributions:
trace_fig = az.plot_trace(trace, var_names=[ 'KIC', 'ft','ϵ'], figsize=(12, 15)); # get and change x labels for i in range(trace_fig.shape[0]): for j in range(trace_fig.shape[1]): if j==0: x_labels = [str(np.round(x,3)) for x in trace_fig[i][j].get_xticks()]; trace_fig[i][j].set_xticklabels(x_labels, fontsize=14); if j==1: y_labels = [str(np.round(y,3)) for y in trace_fig[i][j].get_yticks()]; trace_fig[i][j].set_yticklabels(y_labels, fontsize=14); x_labels = [str(int(x)) for x in trace_fig[i][j].get_xticks()]; trace_fig[i][j].set_xticklabels(x_labels, fontsize=14); title=trace_fig[i][j].get_title() trace_fig[i][j].set_title(title, fontsize=20); trace_fig[0][0].set_title(r'K$_{IC}$', fontsize=20); trace_fig[0][1].set_title(r'K$_{IC}$', fontsize=20); trace_fig[1][0].set_title(r'$f_{t}$', fontsize=20); trace_fig[1][1].set_title(r'$f_{t}$', fontsize=20);
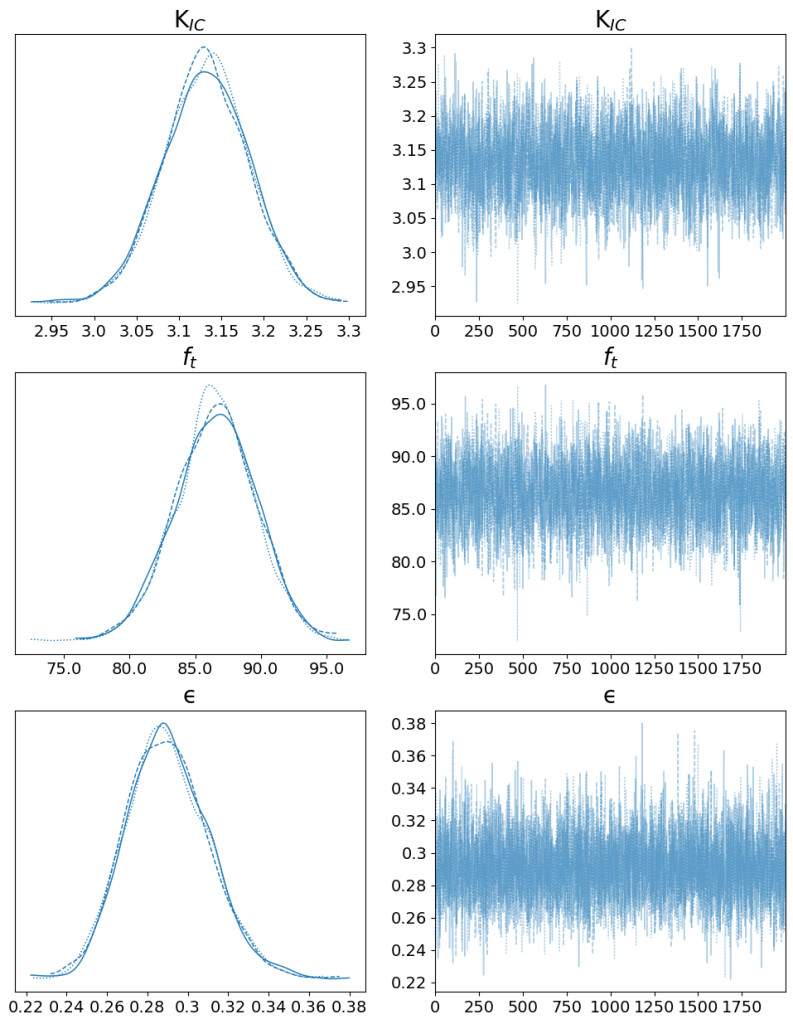
The posterior distribution can be plotted in better quality
# Plot the posterior distribution for KIC az.plot_density(trace, var_names=['KIC'], shade=0.3, hdi_prob=0.99,colors='lightpink') plt.xlabel(r'K$_{IC}$ (MPa m$^{0.5}$)') plt.title('Posterior Distribution for K$_{IC}$') plt.show() az.plot_density(trace, var_names=['ft'], shade=0.3, hdi_prob=0.99) plt.xlabel(r'f$_{t}$ (MPa)') plt.title('Posterior Distribution for f$_{t}$') plt.show() az.plot_density(trace, var_names=['ϵ'], shade=0.3, hdi_prob=0.99,colors='lightgreen') plt.xlabel(r'ϵ (MPa m$^{0.5}$)') plt.title('Posterior Distribution for ϵ') plt.show() # Summarize the results summary = az.summary(trace) print(summary)
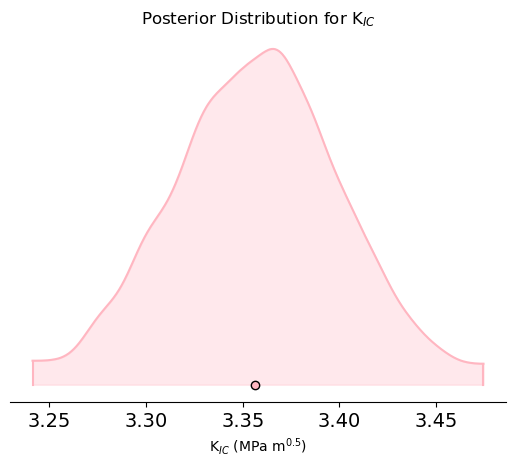
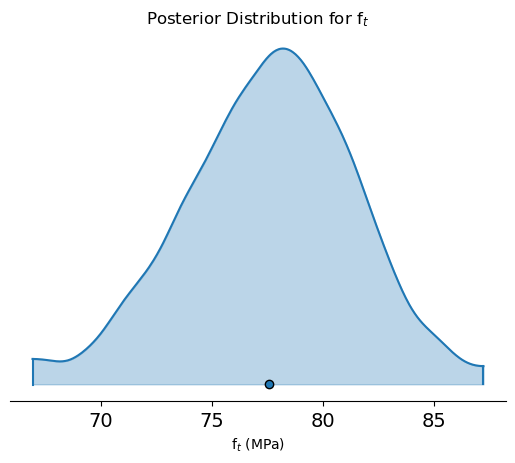
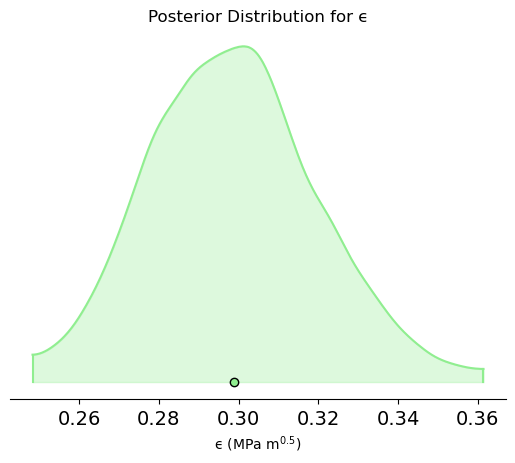
The parameter region that fits the experimental results can be studied with the next code
az.plot_pair(trace,kind='kde', var_names=[ 'KIC', 'ft']) plt.ylabel(r'$\sigma_{C}$ (MPam)') plt.xlabel(r'K$_{IC}$ (MPam$^{0.5}$)')
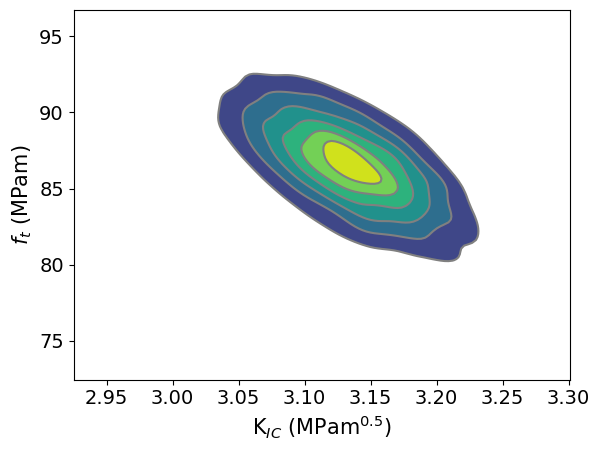
# Posterior samples posterior_KIC = trace.posterior['KIC'].values.flatten() posterior_ft = trace.posterior['ft'].values.flatten() KICmean = trace.posterior['KIC'].mean().values ftmean = trace.posterior['ft'].mean().values fit_KN = KN_func(KICmean,ftmean, radio_vect) # Select some posterior samples for plotting num_samples = 500 # Number of samples to plot posterior_samples_idx = np.random.choice(len(posterior_KIC), num_samples, replace=False) # Plot original data vs. posterior curves plt.scatter(radio_vect, observed_KN_values, color='blue', label='Observed KN data') for i in posterior_samples_idx: plt.plot(radio_vect, KN_func(posterior_KIC[i], posterior_ft[i], radio_vect), color='gray', alpha=0.1) # Plot the true KN curve plt.plot(radio_vect, fit_KN, 'r--', label='Fitted curve', linewidth=2) plt.xlabel('Sample') plt.ylabel(r'K$_N$ (MPa m$^{0.5}$)') plt.ylim([0,None]) plt.legend() plt.show()
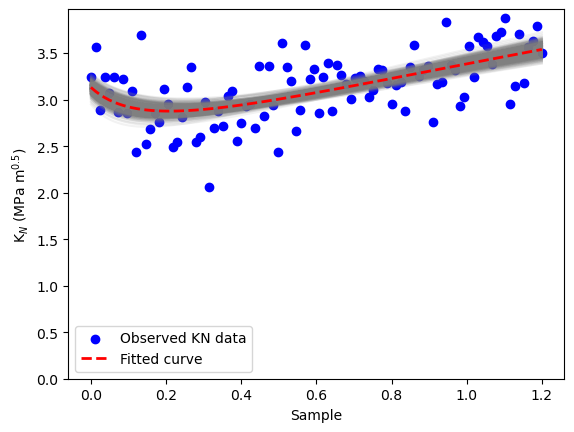